Papers Relating to Bitcoin and Related Subjects in Law: Part XI
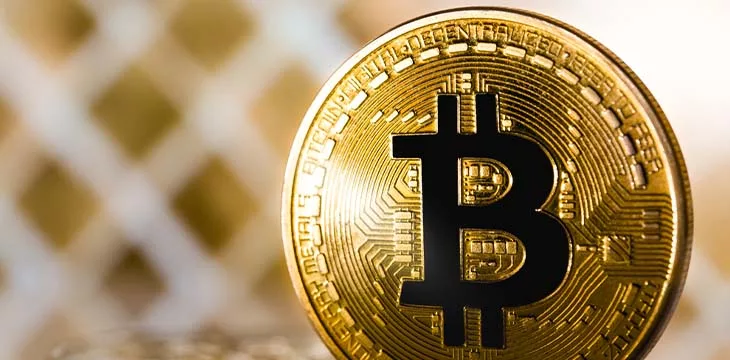
This article was first published on Dr. Craig Wright’s blogand we republished with permission from the author. Read Part 1, Part 2, Part 3, Part 4, Part 5, Part 6, Part 7, Part 8, Part 9 and Part 10.
Measuring the distance between nodes has been considered complex (Chen et al., 2020) and may lead to errors or listed data processing. Due to the problems of measuring networks, some authors have only captured and produced representations of a graph network, without analyzing the effect of power and the influence of each node. A limited approach of this form has been performed on the Ethereum network, providing a model of computing systems on the network (Kim et al., 2018); nevertheless, such an approach fails to distinguish the validity of each node or its influence on other parts of the network.
Fei (2018) addresses some issues in analyzing network influence not addressed by other authors, such as Kim et al. (2018). The new approach to identifying influential nodes in complex networks also summarizes other existing centrality measures, providing a means to capture the intensity and mutual attraction that can exist between nodes on a distributed network like Bitcoin. Through such a process, the author provides a means of comparing the influence of each node, allowing researchers to analyze the comparative power or effect that each system maintains.
Through such an analysis, Fei (2018) provides a means to isolate the central systems and network factors that form giant node components within complex networks, while Chen et al. (2020) discuss automated machine learning technologies that can simplify some of the tasks. Unfortunately, many authors, including Kim et al. (2018), continues to focus on the volume of nodes, ignoring the individual effect that more influential nodes have over the rest of the network.
Annotated bibliography
Chen, D., Lin, Y., Li, W., Li, P., Zhou, J., & Sun, X. (2020). Measuring and Mitigating the Oversmoothing Problem for Graph Neural Networks from a Topological View. Proceedings of the AAAI Conference on Artificial Intelligence, 34(04), 3438–3445. https://doi.org/10.1609/aaai.v34i04.5747
Chen et al. (2020) introduce the concept of graph neural networks (GNNs) as a machine learning model coupled to graph representation. The methodology integrates the mean absolute deviation (MAD) and the smoothness functions of many other neural networks and related machine learning tools. The functionality also allows the capture of network data through an automated process with a toolset known as an adaptive edge optimization system. Finally, the topography measures are analyzed for noise and over-smoothing.
The primary merit of the paper lies in the systematic and quantitative analysis of problems faced by GNNs and the optimization of systems that analyze and capture graph topographies. The analysis has been done on various Pubmed and related public citation networks, and the focus has been on cropping and capturing important information between systems that is not naturally grasped and represented as such.
Fei, L., Zhang, Q., & Deng, Y. (2018). Identification of influential nodes in complex networks based on the inverse square law. Physica A: Statistical Mechanics and Its Applications, 512, 1044-1059. https://doi.org/10.1016/j.physa.2018.08.135
Fei et al. (2018) document the process related to the identification of influential nodes in complex networks. By determining the nodes that have the most influence and are essential for the transmission and broadcasting of traffic, an analysis of decentralization and the interconnection between the systems will be possible. The assignment starts by looking at the various types of centrality measures that already exist, and documenting the algorithm’s shortcomings and limitations. Next, the authors propose using an inverse square law to form an index of mutual attraction between nodes in a complex network.
The article presents a series of experiments and simulations that compare the proposed centrality measure against existing measurements such as closeness centrality, degree centrality and eigenvector centrality. In addition, methods used with online systems such as Google (NASDAQ: GOOGL) in page ranking and leader ranking are also analyzed. Finally, the model and process extend to look at epidemiological systems, including susceptible, infected models. The experimental validation shows good statistical power in the proposed methodology.
Kim, SK, Ma, Z., Murali, S., Mason, J., Miller, A., & Bailey, M. (2018). Measurement of Ethereum Network Peers. Proceedings of the Internet Measurement Conference 2018, 91-104. https://doi.org/10.1145/3278532.3278542
Kim et al. (2018) propose a methodology for measuring network peers in the Ethereum network. The paper begins by introducing and documenting the “smart contract” capability of Ethereum, referring to the system as a “cryptocurrency.” It then presents the argument that Ethereum is the first Turing-complete blockchain system, ignoring the possibilities in Bitcoin. The method is based on the discovery of nodes, and is implemented using a developed tool called NodeFinder. The authors claim that the tool has found over 10,000 nodes by exploring Ethereum’s P2P ecosystem.
There is no analysis of the node functionality, such as the development of blocks, and the measurement and validation focus on finding all the participants in the system. The argument is presented that the tool is validated through external measurements where the node finds other systems that are part of the peer ecosystem. Unfortunately, no information about the creation of blocks has been published. Likewise, no information about the broadcast or transmission of either blocks or transactions is included in the study. The analysis of “non-productive peers” (2018, p. 99) is therefore of little use. Additionally, since the authors have not differentiated between nodes that are clients of the network and nodes that are actively producing blocks and transferring information, the overall value of the paper is limited.
Additional references
Chen, D., Lin, Y., Li, W., Li, P., Zhou, J., & Sun, X. (2020). Measuring and Mitigating the Oversmoothing Problem for Graph Neural Networks from a Topological View. Proceedings of the AAAI Conference on Artificial Intelligence, 34(04), 3438–3445. https://doi.org/10.1609/aaai.v34i04.5747
Fei, L., Zhang, Q., & Deng, Y. (2018). Identification of influential nodes in complex networks based on the inverse square law. Physica A: Statistical Mechanics and Its Applications, 512, 1044-1059. https://doi.org/10.1016/j.physa.2018.08.135
Kim, SK, Ma, Z., Murali, S., Mason, J., Miller, A., & Bailey, M. (2018). Measurement of Ethereum Network Peers. Proceedings of the Internet Measurement Conference 2018, 91-104. https://doi.org/10.1145/3278532.3278542
This article has been lightly edited for clarity.
See: Dr. Craig Wright’s keynote: A Better Internet with IPv6 and BSV Blockchain
New to Bitcoin? Check out CoinGeeks Bitcoin for beginners section, the ultimate resource guide for learning more about Bitcoin – as originally envisioned by Satoshi Nakamoto – and blockchain.