Extend fraud detection with generative AI
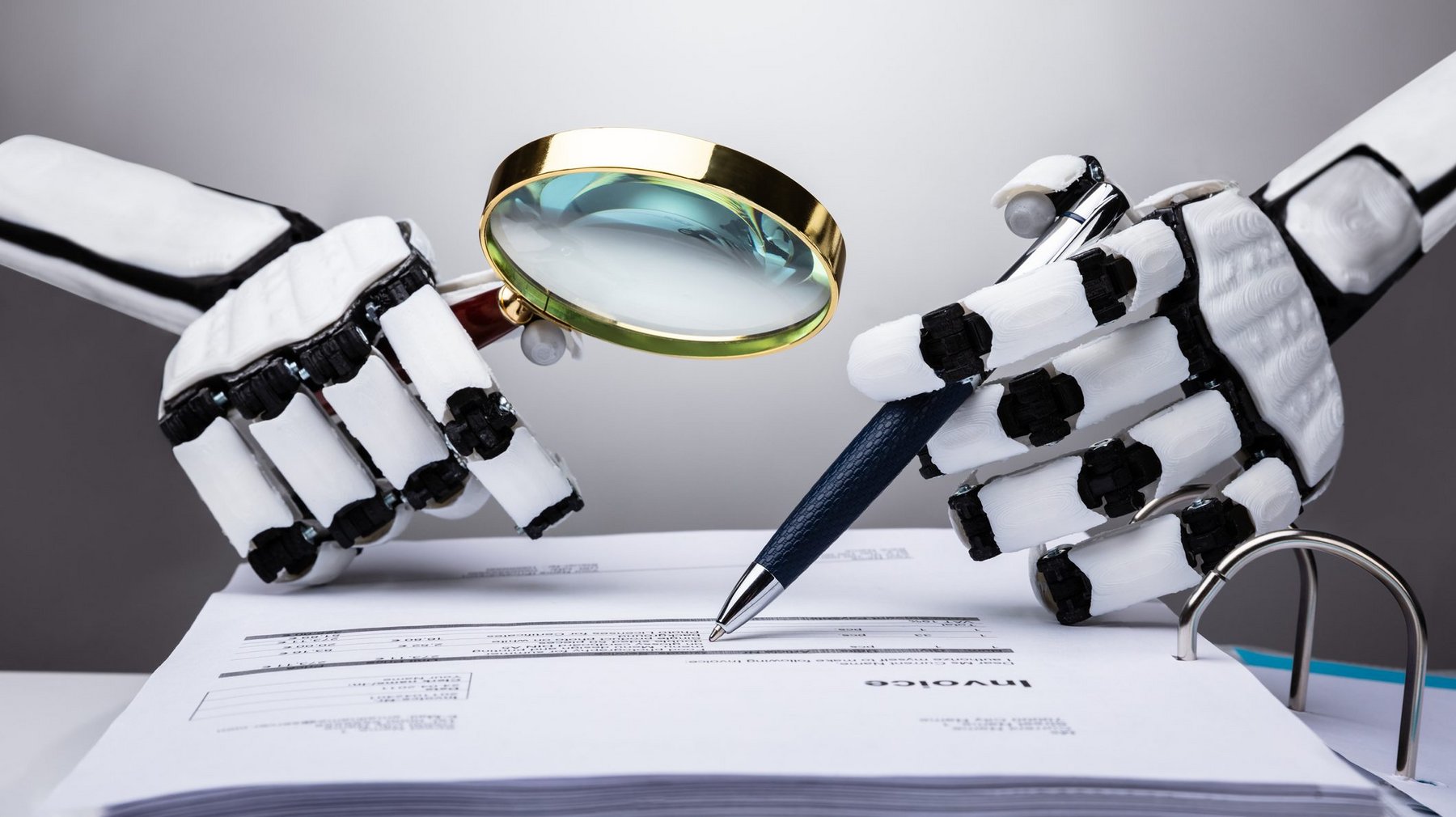
The possible applications of generative AI have been explored by many in recent weeks, but a key topic that has not been fully explored is how data created by generative AI can be used to extend and improve fraud detection strategies, and the implications of using synthetic data to train fraud models and improve detection rates.
It is well known in data science circles that the quality of data presented to a machine learning model makes or breaks the end result, and this is especially true for fraud detection. Many machine learning tools used to detect fraud rely on a strong fraud signal—typically lower than 0.5% of the data—making any model difficult to train effectively. In an ideal data science exercise, the data used to train any AI model would contain a 50/50 mix of fraud/non-fraud samples, but this is difficult to achieve and may therefore be unrealistic for many. Although there are many methods to deal with this (class) imbalance, such as clustering, filtering or oversampling, they do not fully compensate for an extreme data imbalance between genuine and fraudulent records.
What is generative AI and how is it used?
Generative AI, the use of transformative deep neural networks such as OpenAI’s ChatGPT, is designed to produce sequences of data as output and must be trained using sequential data, such as sentences and payment history. This differs from many other methods, which produce simple ‘classifications’ (fraud/non-fraud) based on presented input and training data, which can be presented to the model in any order; a generative AI’s output can continue indefinitely, while classification methods tend to produce single outputs.
Generative AI is therefore the ideal tool for the synthetic generation of data that is based on real data, and the development of this technology will have important applications in the fraud detection domain, where, as previously highlighted, the amount of viable fraud samples is very low and difficult for a machine learning to learn effectively of. With generative AI, a model can use existing patterns and generate new, synthetic samples that are like “real” fraud samples, increasing the fraud signal for core fraud detection tools.
A typical fraud signal is a combination of genuine and fraudulent data. The real data will (usually) come first in the sequence of events and contain real behavioral activity of a cardholder, for example with fraudulent payments mixed in when the card/other payment method is compromised. Generative AI can produce similar payment sequences, simulating a fraud attack on a card, which can then be added to training data to help machine learning fraud detection tools and help them perform better.
How can generative AI help detect fraud?
One of the biggest criticisms of OpenAI’s ChatGPT is that current models can produce inaccurate or “hallucinogenic” output – a flaw that many in the payments and fraud space are rightly concerned about, as they don’t want their public tools like customer service chatbots present false or fabricated information. However, we can take advantage of this “bug” to generate synthetic fraud data, as synthetic variation in synthesized output can generate completely unique fraud patterns, enhancing the fraud detection performance of the final fraud defense model.
As many will know, repeated examples of the same fraud signal do not effectively improve detection, as most machine learning methods require very few instances of each to learn from. The variation in generated output from the generative model provides robustness to the final fraud model so that it can not only detect the fraud patterns present in the data, but also detect similar attacks that could easily be missed using a traditional process.
This can be somewhat alarming for cardholders and fraud managers – who are right to ask how a fraud model trained on fabricated data can help improve fraud detection, and what the benefits of doing so might be. What they may not realize is that before any model is applied to direct payments, it goes through rigorous evaluation exercises to ensure expected performance. If the model does not meet the extremely high standards expected, it is discarded, and replacements are trained until a suitable model is found. This is a standard process and one that is followed with all machine learning models produced, as even models trained on authentic data can end up delivering substandard results at the evaluation stage.
Generative AI is a fascinating tool with many applications across a variety of industries, but current iterations, however clever, have their problems. Fortunately, the features that are seen as very serious problems for some industries are important features for others, but the requirement for strict regulation and governance remains. Future use of generative AI requires a full review of how models trained on partially generated data are used, and governance processes should be tightened accordingly to ensure that the required behavior and performance of the tools are consistently met.